Understanding Risk Models in Insurance: A Detailed Overview
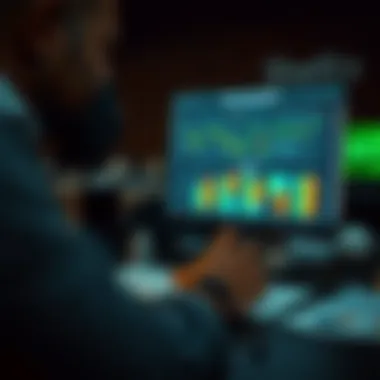
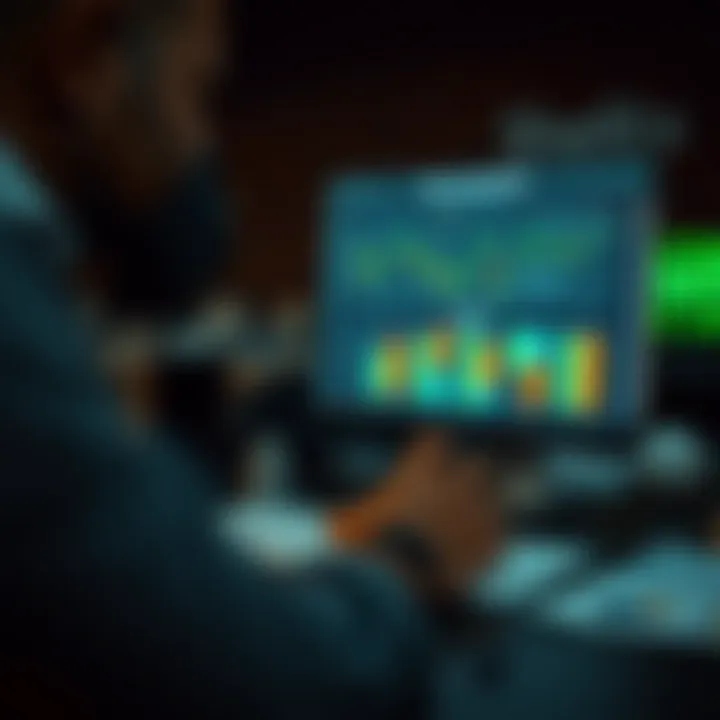
Intro
In the world of insurance, understanding risk is fundamental. Risk models serve as the backbone, offering insurers insights into pricing policies and developing strategies that balance protection and profitability. As the landscape evolves, these models become increasingly sophisticated, incorporating big data analytics and machine learning techniques that enhance predictive capabilities. The intent of this article is to unravel these intricacies, providing both newcomers and seasoned industry veterans with a roadmap to navigate the complex but rewarding terrain of risk modeling.
Investment Terminology
Understanding the language used in risk modeling is crucial for grasping its concepts. This section will furnish you with key terms and definitions that you’ll encounter frequently.
Key Terms and Definitions
- Risk: The likelihood that an insured event will occur causing loss.
- Premium: The amount paid periodically by the insured to enjoy coverage against risks.
- Underwriting: The process of evaluating risk and determining the terms and pricing of coverage.
- Loss Ratio: A measurement of the losses in relation to the earned premiums, an essential figure for gauging an insurer's profitability.
- Actuarial Science: A discipline that uses mathematical and statistical methods to assess risk in insurance and finance.
These terms lay the foundation for deeper exploration into risk models and highlight the critical role they play in decision-making processes.
Common Acronyms in Insurance
- ROI: Return on Investment
- IA: Insurance Agent
- NALC: National Association of Letter Carriers
- RFC: Request for Change
- ISO: Insurance Services Office
Understanding these acronyms can smooth the learning curve and foster better communication within the industry.
Expert Insights
Incorporating insights from industry experts can lend additional perspective on how risk models are applied within real-world scenarios.
Investment Strategies Overview
Risk models influence various investment strategies by helping portfolio managers assess potential hazards connected to different financial products. By employing statistical models, these managers can weigh the expected returns against risks, allowing for informed diversification decisions. This approach helps insurers selectively engage in markets that align with their risk appetite, enhancing long-term sustainability.
Tips from Financial Advisors
Financial experts often stress the importance of understanding insurance products and their underlying risks. Some crucial tips include:
- Stay Informed: Regularly update yourself on emerging trends in data analytics and risk assessment methodologies.
- Ask Questions: Don’t hesitate to seek clarification on policy terms and conditions, as these can greatly affect your coverage.
- Utilize Technology: Leverage reputable analytics tools to gain insights into risk models that may be relevant for personal or business insurance.
By internalizing these insights, both insurers and policyholders can foster a more proactive approach toward managing risk, ultimately leading to more informed decisions.
A robust understanding of risk models not only equips insurers with the tools to evaluate potential losses but also enhances their ability to protect policyholders effectively.
The journey doesn’t stop here; join us as we delve deeper into specific methodologies and real-world applications of these risk models.
Foreword to Risk Models in Insurance
In today's complex landscape of the insurance industry, risk models serve as the backbone of effective decision-making. These models help insurers evaluate and quantify potential losses, providing a systematic way to understand and manage uncertainties inherent in underwriting and claims processing. Without such frameworks, insurers would be akin to sailors navigating uncharted waters without a compass—vulnerable to unforeseen challenges and miscalculations.
Understanding risk models is not merely about grasping underlying statistics; it encompasses the very essence of insurance itself—protection against uncertainties. For both emerging investors and seasoned financial enthusiasts, familiarizing themselves with how these models operate is paramount.
Among the several benefits that come from utilizing risk models include:
- Informed Pricing Strategies: Insurers can develop premium structures that accurately reflect risk levels, ensuring profitability while maintaining competitive rates.
- Enhanced Customer Trust: When clients understand how their premiums are evaluated, they can have greater confidence in their insurers.
- Thoughtful Resource Allocation: Companies can better predict claims and allocate reserves, directly influencing their operational efficiency.
The importance of risk models also lies in their adaptability. As external factors—such as economic conditions or emerging technologies—shift, risk models can be updated or modified, ensuring that insurers remain proactive rather than reactive. This ongoing evolution is crucial in an industry that constantly grapples with new challenges.
As we delve deeper, attention will be given to how these risk models have been shaped by historical context, data reliance, and emerging technologies, equipping readers with a thorough understanding of risk modeling in insurance.
Definition and Purpose of Risk Models
Risk models are quantitative tools that help insurers estimate the likelihood of an event and the potential financial impact it may have. Essentially, these models draw from historical data and established mathematical principles to predict future events.
At their core, the purpose of risk models is to enable better decision-making. Insurers utilize them to:
- Determine premium rates for different types of policies.
- Assess claim probabilities and potential payouts.
- Identify trends and patterns in claims data, which can lead to enhanced risk management strategies.
In addition to financial forecasting, risk models also aid in regulatory compliance and ensure that insurers maintain sufficient reserves to cover potential claims, ultimately promoting long-term sustainability.
Historical Context of Risk Assessment
The concept of risk assessment in insurance can be traced back centuries. While today’s models are highly sophisticated, the foundational idea of evaluating risk has deep roots. From ancient maritime trade, where shipowners would share their risk through collective agreements, to the establishment of life insurance in the 17th century, the principles of risk management have evolved significantly.
In the early days, assessment relied heavily on anecdotal evidence and subjective judgment. However, with industrialization and the advent of statistics, insurers began to apply mathematical principles to their assessments. The introduction of probabilistic risk analysis in the 20th century marked a pivotal moment, allowing for more structured and reliable models.
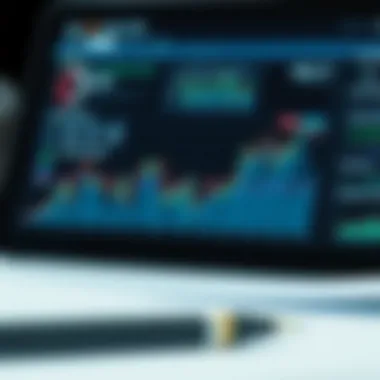
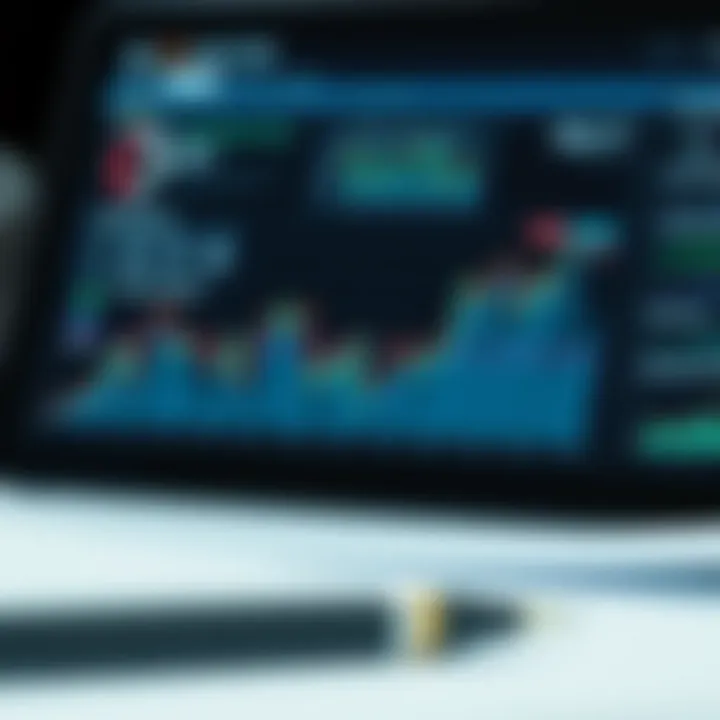
The digital revolution further transformed risk assessment by enabling the collection and processing of vast amounts of data. The ability to analyze trends over time has given rise to predictive modeling, which seeks not only to assess current risk but also to forecast future scenarios. As such, the journey from rudimentary assessments to today's complex algorithms illustrates the increasing importance of sophisticated risk modeling in ensuring the stability and effectiveness of the insurance industry.
The Role of Data in Risk Modeling
Data underpins the entire framework of risk modeling in the insurance sector. Its importance cannot be overstated, as it shapes the strategies and methodologies exemplified in the risk assessment process. Without a solid foundation of data, insurers would be venturing into the unknown, making decisions that could potentially lead to losses when assessing risks. The role of data is multifaceted, encompassing various types, influencing accuracy and reliability, and ultimately steering the effectiveness of the risk models employed.
Types of Data Used
Claims Data
Claims data serves as a cornerstone in the insurance industry, encapsulating information about past claims made by policyholders. This data is particularly valuable because it reveals patterns and trends that can inform risk assessments. One key characteristic of claims data is its focus on historical performance, allowing insurers to gauge which types of claims occur under specific circumstances. It is a beneficial choice as it is often readily accessible and encompasses a wealth of real-world insights. This leads to more accurate predictions in both individual risk assessments and pricing strategies.
However, there's a unique feature regarding claims data: it can reflect both low-frequency, high-severity risks and high-frequency, low-severity claims. While it helps pinpoint frequent minor disasters, it may also obscure rarer events that have catastrophic potential. Thus, although claims data provides critical insights, it must be viewed with caution, ensuring that all risks are adequately represented.
Policyholder Information
Policyholder information includes demographic details, such as age, gender, occupation, and location, which play a critical part in risk assessment. By analyzing these factors, insurers can understand how different characteristics correlate with risk likelihood. This type of data is popular because it allows for tailored services to policyholders based on their unique profiles.
The unique feature of policyholder information lies in its ability to segment the market effectively. If, say, young drivers with less experience are statistically more likely to file claims, insurers can adjust their policies accordingly. Moreover, its use leads to personalized premiums, which enhances customer satisfaction.
Yet, relying too heavily on such information can raise ethical questions about discrimination. It’s crucial for insurers to strike a balance, ensuring fairness while also safeguarding business interests.
External Data Sources
External data sources encompass a variety of information that exists outside of direct insurance company records. This can include credit scores, social media activity, and even geographical data like crime rates. The increasing availability of such data has made it an invaluable asset in modern risk modeling practices.
One key characteristic of external sources is their timeliness. Unlike historical claims that take time to accumulate, external data often provides up-to-date insights that can sharpen an insurer's competitive edge in the market. By leveraging this information, insurers can preemptively adapt to changing risk landscapes, making it possible to set prices not just based on past events, but projected future ones.
However, there are advantages and disadvantages to using external data. While it can enhance prediction accuracy, its reliability varies significantly. Furthermore, there are privacy concerns regarding how this information is sourced and used. Incidents of data breaches have raised alarms, prompting regulatory scrutiny and necessitating transparent practices in data handling.
Data Quality and Its Impact
Data quality emerges as a critical linchpin in the risk modeling process. In an era where data-driven decision-making dominates, ensuring accuracy, completeness, and consistency is paramount. If the data fed into risk models is riddled with errors, the output will be just as flawed, leading to mispriced premiums or poorly assessed risks.
Insurers must implement robust data governance measures, emphasizing a cycle of continuous improvement in data management. The impact of high-quality data is evident across the spectrum, from enhanced decision-making processes to improved stakeholder trust. Thus, investing in high-quality data can yield significant returns, positioning insurers to navigate the sometimes-turbulent waters of risk analysis with greater precision and confidence.
Types of Risk Models
The realm of insurance is a complex landscape defined by uncertainty and potential hazards. Understanding the different types of risk models is crucial as these models serve as the backbone for decision-making processes in the industry. They provide insurers with valuable insights into the likelihood of claims, allowing for more informed pricing and underwriting strategies. Delving into these models enhances comprehension of how insurance companies assess risks, paving the way for improved service delivery and profitability.
Statistical Risk Models
Statistical risk models form the foundational layer of risk assessment in the insurance sector. These models rely heavily on historical data, employing statistical methods to predict future outcomes. For instance, insurers often utilize logistic regression to determine the likelihood of a claimant submitting a fraudulent claim based on various indicators like claim size and timing.
The importance of statistical models lies in their objective approach to risk evaluation. They strip away subjective biases and facilitate uniformity in risk assessment across different portfolios. Moreover, these models help in benchmarking claims data against broader industry trends. This not only aids in accurate pricing of insurance policies but also assists in forecasting future claims.
Predictive Analytics in Insurance
Predictive analytics is transforming how risk models are utilized in insurance. It goes beyond traditional statistical methods, leveraging advanced analytics to uncover patterns within data that may not be immediately apparent. Insurers use predictive analytics to refine their risk models, directly impacting their portfolio management strategy.
For example, through predictive modeling, insurers can identify high-risk applicant segments that require closer scrutiny or adjustment in policy terms. They can even forecast how economic shifts might influence claims frequency. The growing importance of predictive analytics lies in its capacity to adapt quickly to changing market conditions—making it a linchpin in modern risk assessment.
Machine Learning Applications
Machine learning has carved its niche in risk modeling, offering sophisticated algorithms that learn from data without being explicitly programmed. This is where dynamic adjustment to risk models comes into play, allowing insurers to fine-tune their approach continuously as new data rolls in.
Supervised Learning
Supervised learning is a type of machine learning where an algorithm is trained on a labeled dataset, enabling it to predict outcomes based on past experiences. Its contribution to risk modeling is significant as it improves accuracy in predicting claim outcomes. A key characteristic of supervised learning is its ability to handle a multitude of variables, making it particularly popular in scenarios like predicting the likelihood of policyholder defaults.
There’s a unique feature in supervised learning where the model can be validated with a separate test dataset, ensuring that it's not merely memorizing the input data but genuinely learning patterns. Although it often yields strong results, supervised learning can sometimes fall short in scenarios where labeled data is scarce or when dealing with highly volatile environments.
Unsupervised Learning
Contrasting with its supervised counterpart, unsupervised learning analyzes data without pre-existing labels. This allows it to identify hidden patterns or groupings among data, which can be particularly beneficial for uncovering new risk categories or assessing unusual claim trends.
A key characteristic of unsupervised learning is its flexibility in discovering insights without requiring a specific outcome to guide it. This methodology offers a unique vantage point, especially for insurers looking to innovate in their risk assessments. However, the lack of labeled data can result in difficulties in interpreting the results precisely, making implementing changes based on these models a more challenging prospect.
"The shift toward machine learning applications signifies a notable evolution in how insurers handle risk, highlighting the need for continuous learning and adaptation."
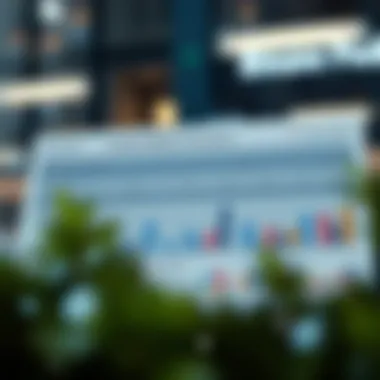
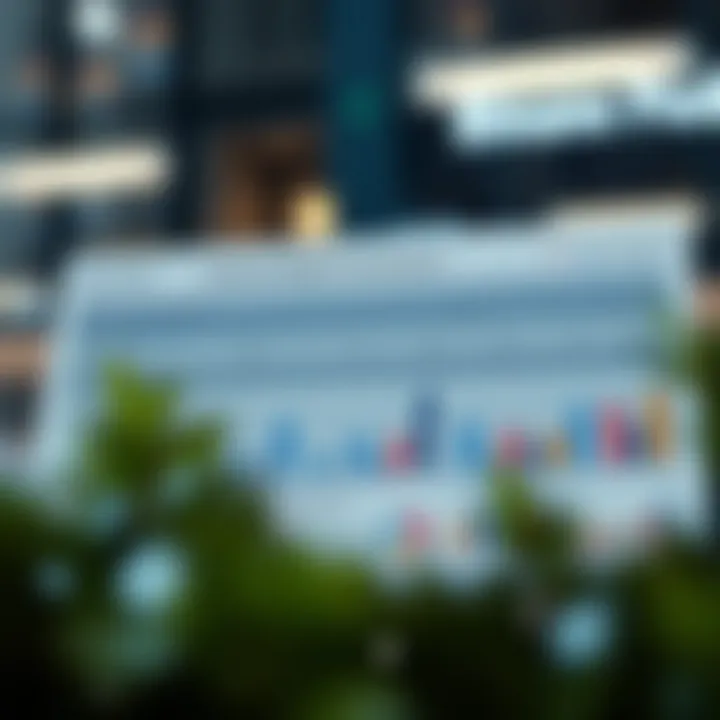
In summary, understanding these various types of risk models—not only illuminates their individual merits but also emphasizes how combining them can lead to a more comprehensive risk assessment. With the landscape ever-changing, being at the forefront of these innovations ensures a competitive edge in the marketplace.
Risk Assessment Process
Risk assessment is the bedrock of any effective risk management strategy in the insurance sector. The complex nature of risks not only demands careful navigation through possible uncertainties but also requires a structured approach to evaluate and understand them comprehensively. This process aids insurers in determining the right amount for premiums, designing policies, and ultimately, it shapes the financial future for both insurers and policyholders alike.
Identifying Risks
The first step in the risk assessment process is identifying risks. This stage serves as the foundation upon which all other risk management tasks are built. Risks can emerge from various sources, such as environmental factors, market fluctuations, and even human behaviors. Each insurance product comes with its unique set of risks. For example, auto insurance requires assessing roadside hazards while health insurance might focus on lifestyle choices affecting health.
- Internal Factors: Company operation, employee practices, and financial stability.
- External Factors: Economic trends, regulatory changes, natural disasters.
A thorough assessment can involve using tools like risk matrices, surveys, or industry reports to pinpoint uncertainties accurately. Identifying risks allows insurers to create tailored solutions for their clientele.
Evaluating Risks
The next phase is evaluating the identified risks. Here, the focus shifts to understanding the likelihood and potential impact of each risk. Insurers typically assess risks based on quantitative and qualitative methods. For example, they might analyze past claims histories to calculate the probability of future incidents.
Moreover, understanding the severity of risks enables companies to prioritize which risks require immediate action and which can be monitored over time.
Key considerations in evaluating risks include:
- Frequency: How often does a particular risk occur?
- Severity: What costs or damages accompany this risk when it does occur?
Effective risk evaluation can lead to more informed decision-making. It positions insurers to set more precise pricing and establish loss reserves that safeguard their financial health.
Mitigating Risks
Finally, the process culminates in mitigating risks, a crucial step in the overall risk management framework. Once insurers identify and evaluate risks, they need to employ strategies to minimize their exposure. Various mitigation tactics can be adopted, such as implementing stricter underwriting guidelines, diversifying the insurance portfolio, or even employing technology to enhance risk awareness.
- Transfer: Use of reinsurance to transfer part of the risk to another entity.
- Avoidance: Altering business practices to eliminate risk.
- Reduction: Implementing safety measures to lower the chance of a claim.
In a world where uncertainty is a given, the capacity to mitigate risks effectively stands as the driving force behind sustainable insurance practices.
"Safeguarding against potential losses isn’t just prudent; it’s essential for maintaining a resilient insurance operation."
In summary, the risk assessment process is a dynamic and iterative cycle that requires ongoing attention and adjustment, underscoring the necessity for insurers to remain vigilant and adaptable as new risks continue to emerge in an ever-changing landscape.
Application of Risk Models in Pricing
Understanding how risk models apply to pricing is crucial for stakeholders in the insurance industry. These models are not merely theoretical; they are foundational for devising fair and competitive premiums that balance profitability with customer retention. Insurers leverage various methodologies to ensure they can predict future claims accurately, which in turn influences how premiums are structured.
Developing Premium Structures
Developing premium structures is a process that requires a keen analysis of data and risk factors. When insurers craft a premium, they take into account a multitude of variables that correlate with the likelihood of a policyholder making a claim. For instance, someone living in a flood-prone area will often face higher premiums than someone in a region with low natural disaster risk.
- Risk Evaluation: Each risk is evaluated based on both historical data and predictive analytics. Insurers may look into statistical models that highlight trends, such as claims frequency and severity in similar demographics.
- Actuarial Science: Actuaries utilize intricate calculations to assess risks and set premiums accordingly. This profession applies mathematical principles to evaluate risk and ensure the company remains solvent while providing insurance coverage.
- Customization: Tailoring premiums to individual policyholders is increasingly becoming common. Personalized pricing takes into account unique factors such as driving history in auto insurance or health metrics in health insurance. This approach serves two purposes: it minimizes adverse selection and enhances customer satisfaction.
By developing robust premium structures, insurers can not only meet regulatory requirements but also establish fair pricing that reflects the policyholder's real risk.
Adapting to Market Changes
The landscape of the insurance market is evolving, and the ability to adapt to changes is vital for success. Risk models play a critical role in helping insurers pivot according to market demands and economic shifts. This flexibility can dictate the survival of an insurer in a competitive marketplace.
- Risk Trends: Emerging trends, such as the rise of telematics in auto insurance, demand that insurers quickly adjust their pricing structures. By incorporating real-time data, insurers can assess how driving behavior impacts risk profiles more dynamically than ever before.
- Economic Influences: Fluctuations in the economy, such as inflation, can lead to a need for adjustments in pricing. Risk models consider these external factors to ensure premiums remain viable and competitive. For example, if repair costs for vehicles surge due to supply chain issues, insurers must accordingly increase premiums to maintain operational health.
- Regulatory Changes: Changes in regulations can also necessitate a reevaluation of pricing strategies. Insurers must be aware of legal requirements, such as minimum coverage levels or changes in industry standards, and adapt their pricing structures to remain compliant.
Adapting to market changes is not just about survival but about strategic positioning. Insurers that effectively use risk models to make timely adjustments can reap benefits that extend beyond mere compliance—finding a balance between competitive pricing and sustainable growth.
Summary: The integration of risk models in pricing not only fosters fairness in premium development but also equips insurers to stay ahead in an ever-changing market. Understanding these dynamics offers valuable insights for both providers and consumers alike.
Case Studies in Risk Modeling
Understanding risk modeling isn't merely about theoretical concepts; it also requires grounding in real-world applications. By analyzing various case studies, we can uncover how risk models operate in practice, highlighting both their strengths and limitations. The insights gleaned from these case studies furnish valuable lessons for industry players, ensuring that both insurers and policyholders make informed decisions. They also illustrate the benefits of adaptive strategies and the importance of learning from historical and current experiences.
Private Sector Innovations
In the private sector, case studies showcase numerous innovative practices that reflect the ever-evolving landscape of risk modeling. One notable example is the use of advanced machine learning algorithms by companies like Progressive Insurance. These algorithms analyze data from telematics—devices in cars that monitor driving behavior—to assess risk more accurately.
By collecting real-time data, Progressive can offer personalized premiums based on actual driving patterns rather than generalized risk models. This approach has been a game changer, allowing clients who drive safely to reap significant financial benefits while also promoting better driving habits.
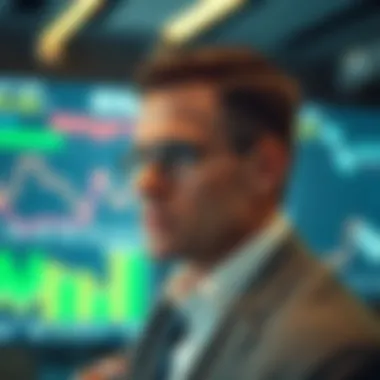
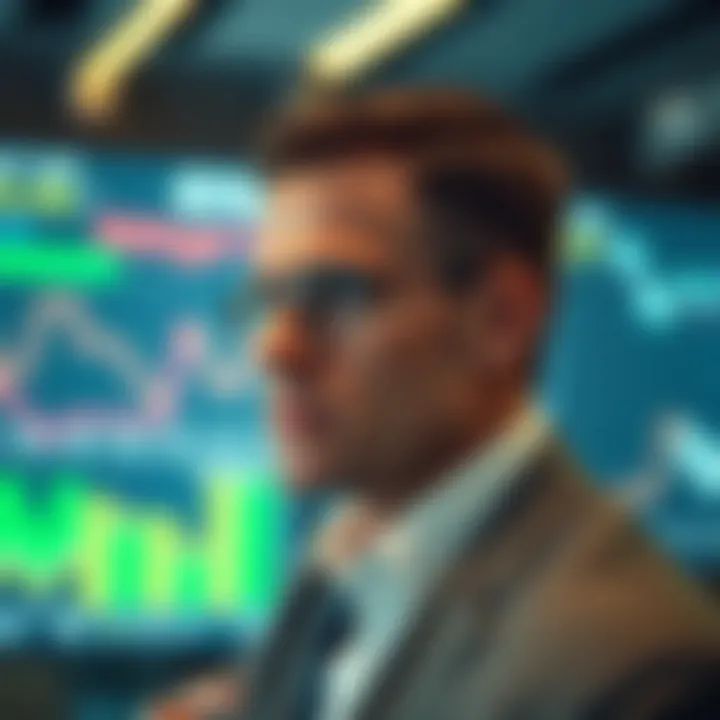
- Advantages:
- Costs are reduced for safe drivers.
- Increased customer satisfaction.
- Potential reduction in claims due to improved driving behavior.
This case exemplifies how technology not only helps in pricing but also takes a proactive stance on minimizing risk by nudging drivers towards safer habits. However, it also raises questions on data privacy, as the collection and use of behavioral data can lead to significant concerns among consumers.
Public Sector Applications
Public sector applications of risk models highlight the importance of guiding larger populations rather than just individual consumers. A prominent example here is found in public health insurance programs like the National Health Service (NHS) in the United Kingdom. The NHS employs risk models to determine funding needs across regions, assessing varied factors like demographic health statistics and socio-economic conditions.
By applying sophisticated actuarial methods, the NHS can distribute resources to areas that show higher health risks, ultimately striving for equitable healthcare access. This case illustrates how risk modeling can serve the public good, aiming to optimize resource allocation for better overall community outcomes.
- Key Considerations:
- Ensures that regions with higher health risks receive adequate support.
- By analyzing trends, health officials can anticipate healthcare needs over time.
- Fosters a better understanding of how societal factors influence health outcomes.
One downside to using risk models in this way is the potential for data misinterpretation, which can lead to inequities if not handled thoughtfully. Missteps in this arena underscore the need for ongoing scrutiny and improvement of models to ensure they genuinely serve their intended purpose.
"Case studies in risk modeling are not mere stories; they are critical reflections that guide the ongoing evolution of our understanding and implementation of risk assessment."
In summary, case studies in risk modeling—both in the private and public sectors—offer crucial insights that promote better decision-making and innovative practices. The lessons learned help bridge the gap between theoretical applications and real-life impacts, informing future strategies and advancements in the insurance industry.
Challenges in Risk Modeling
Risk modeling in insurance isn’t just about crunching numbers. It’s a delicate balance between art and science, navigating through a myriad of challenges that can make or break an insurance company's strategy. This section discusses why understanding these obstacles is crucial. The realm of risk modeling often intersects with sensitive areas, including data privacy, regulatory compliance, and the ever-looming demand for algorithmic transparency. Addressing these concerns doesn’t just protect insurers; it fosters trust among policyholders.
Data Privacy Concerns
In the age of big data, the term data privacy rings alarm bells. Insurance companies thrive on collecting extensive data about their policyholders—this includes everything from basic demographics to intricate social behaviors. As they dive deeper into modeling risks, ensuring the sacredness of this data becomes paramount.
A well-known real-life example is the backlash faced by companies like Equifax when data breaches expose sensitive customer information. Such incidents underscore the dire need for robust data protection mechanisms. Insurers must walk a tightrope, striving for a granular understanding of risk without overstepping privacy boundaries. This is vital not just for regulatory reasons, but also for maintaining customer trust. Although it can feel burdensome, putting strict data governance policies in place prepares companies to face audits and public scrutiny.
- Key Considerations:
- Implementing rigorous data encryption.
- Using anonymization techniques in data modeling.
- Educating employees about data handling practices.
Future Directions of Risk Modeling
The landscape of risk modeling within the insurance industry is rapidly shifting, thanks mainly to technological advancements and evolving data utilization strategies. Companies that keep pace with these changes stand at a significant advantage, enhancing not just their operational efficiency but also improving the accuracy of their risk assessments. This section delves into the foreseeable advancements in risk modeling, particularly highlighting new technologies and the integration of artificial intelligence.
Emergence of New Technologies
The wave of innovation in technology has brought forth tools that fundamentally change how insurers view and assess risk. Companies are now leveraging cloud computing, blockchain, and the Internet of Things (IoT) to gather and analyze data like never before.
Key technologies shaping risk modeling include:
- Cloud Computing: Aiding in storing large volumes of data and providing powerful computing capabilities. This allows for real-time risk assessments based on up-to-date information.
- Blockchain Technology: Enhancing transparency and security in data sharing, thus fostering trust between insurers and their clients while reducing fraud risk.
- IoT Devices: Collecting granular data from natural settings, like telematics in vehicles, which offers insights into individual behaviors and risks.
These technologies not only make it easier to gather data but also enhance the quality of insights derived from it. As insurers adopt these tools, they gain richer, more nuanced views of risks tied to policies.
"Incorporating new technologies in risk modeling doesn't just add tools for analysis—it provides a fresh lens through which we can perceive risk itself."
Integration with Artificial Intelligence
Artificial intelligence (AI) is becoming a cornerstone in the evolution of risk modeling. Its ability to analyze complex datasets, recognize patterns, and predict outcomes is redefining how insurance professionals approach risk.
Some notable aspects of AI in risk modeling include:
- Enhanced Predictive Analytics: AI algorithms can sift through vast amounts of information much faster than traditional models, making it possible to uncover patterns that might otherwise go unnoticed.
- Real-time Adjustments: Instead of static assessments, AI allows for dynamic modeling which changes as new data comes in. This responsiveness is critical in volatile markets or environments where risks are constantly evolving.
- Natural Language Processing: AI tools are increasingly being developed to analyze unstructured data, such as customer feedback or market reports, providing insurers with a more complete picture of risk factors.
As these AI technologies become more sophisticated, we can expect them to lead to more personalized insurance products and pricing. This trend may finally bridge the gap between risk assessment and customer satisfaction, ensuring that policies are tailored to the unique needs of every individual policyholder.
Moving forward, a focus on both emerging technologies and AI integration will not just support existing frameworks but will likely revolutionize how risk is understood in the insurance industry.
Epilogue
From the definition and purpose of risk models to their practical applications in real-world situations, it's clear that these tools hew a path through the complexities of risk assessment. For insurers, these models provide not just a means to estimate potential losses but also a framework to maintain financial health under varying conditions.
Key Points to Remember:
- Risk Models Foster Informed Decision Making: Both insurers and policyholders benefit from risk models, as they enable better understanding and communication of risk.
- Data is the Lifeblood: The quality and type of data used are crucial in shaping accurate risk assessments and predictions.
- Challenges Are Ever-Present: Issues such as data privacy, regulatory compliance, and algorithm transparency remain significant hurdles in the deployment of effective risk modeling frameworks.
The efficacy of risk models hinges on the delicate balance between detailed analysis and practical application, ensuring that they do not just exist as theoretical constructs but rather serve as actionable tools.
As we look toward the future of risk modeling, the emergence of new technologies and the integration of artificial intelligence will likely bring forth not only innovations but also new challenges. Such evolutions are crucial for the adaptation of insurers to a rapidly changing landscape, necessitating a continuous commitment to refining these models.